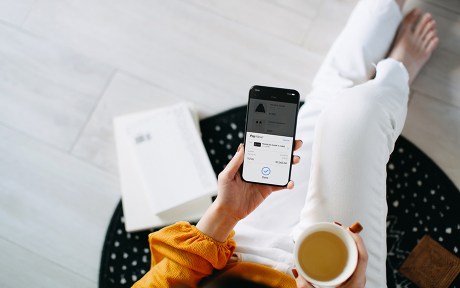
Most American consumers likely are familiar with credit scores, as every lender in the United States uses them to evaluate credit risk. But the Customer Lifetime Value (CLV) that many firms use to target ads, prices, products, and service levels to individual consumers may be less familiar, or the Affluence Index that ranks households according to their spending power. These are just a few among a plethora of scores that have emerged recently, consequence of the abundant consumer data that can be gathered online. Such consumer scores use data on age, ethnicity, gender, household income, zip code, and purchases as inputs to create numbers that proxy for consumer characteristics or behaviors that are of interest to firms. Unlike traditional credit scores, however, these scores are not available to consumers. Can a consumer benefit from data collection even if the ensuing scores are eventually used “against” her, for instance, by enabling firms to set individualized prices? Would it help her to know her score? And how would firms try to counteract the consumer’s response?
Concerns about “Scoring”
A distinguishing feature of these scores is that the data brokers that produce them also sell them to companies for market-segmentation strategies. Thus, these scores do not merely affect a consumer’s interaction with a single firm: the information carried by the score creates links across interactions with different firms and industries over time. The argument in favor is that data collection adds value by creating gains from trade, and scores are a convenient way of packaging data. But adverse welfare effects can arise. For example, if a consumer makes a big purchase, leading her “profitability” score to increase, she may face higher prices tomorrow.
In a recent paper, we developed a model of score-based price discrimination. Our model shuts down any value creation to isolate the mechanisms by which consumers can be harmed by data collection, and the focus on price discrimination stems from the increasingly granular e-commerce targeting and product-steering techniques that make de facto discriminatory pricing a real possibility. In our setup, a consumer interacts with a sequence of firms, and her willingness to pay for the firms’ products is her private information. Because purchases carry information about willingness to pay, and the latter is positively correlated over time, firms use scores that are based on signals of past purchases to set prices. In this context, our analysis examines how consumer welfare is affected by the interplay between different degrees of consumer sophistication (does the consumer know about the scores and the links they create?) and of score transparency (can consumers check their current score?).
Harms and Benefits
Price discrimination unambiguously harms naïve consumers—that is, those who do not recognize the links across transactions—but it can benefit strategic consumers. Specifically, in the naïve case, consumer welfare falls with the quality of the signals available to the firms. Firms in turn are better off. More strikingly, compressing data into a score does not protect consumers at all. This is because firms can aggregate data about purchases in the form of a score belonging to the class that we study, without any loss in predictive power. This class is parametrized by the relevance that each score gives to past signals of behavior, so that a large weight on the past leads to a score with high persistence.
By contrast, a strategic consumer can benefit from the presence of scores even if firms ultimately use them against her, since she can reduce her quantity demanded to manipulate her score. Consider the figure below, depicting a traditional monopoly problem between a consumer with downward-sloping demand and a single firm, say Firm 1. If there is only one interaction, the consumer does not adjust her behavior, resulting in an outcome with Q units purchased at price P. But suppose now that a second firm interacts with the consumer tomorrow after seeing a signal of the first-period purchase. Because the consumer recognizes the impact of her first-period choice on the second period price, she will attempt to reduce Firm 2’s signal—and hence her score—by adopting a lower demand, which reduces her purchases to Q’.
Gains and Losses from Strategic Demand Reduction
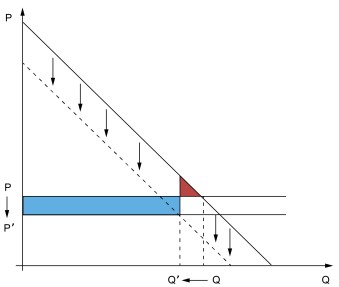
The consumer suffers because she buys less (with the loss represented by the red area). And while not depicted, she also suffers from future price discrimination due to information about her willingness to pay (that is, the intercept of her demand function) getting transmitted to Firm 2. However, Firm 1 is forced to lower its price (P’ in the figure) after the strategic demand reduction occurs. If the consumer has high willingness to pay, the benefit of this discount applied to many units is such that she wants to be tracked (the blue area—a benefit—grows as the intercept of demand increases).
Managing Consumers’ Strategic Response
The strategic demand reduction implies that purchases are less sensitive to changes in willingness to pay. Thus, signals lose informativeness, and price discrimination with scores is less effective. These losses cannot be eliminated: if firms use scores that are best predictors in an ex-post sense, that is, given the available data, strategic consumers will adjust their behavior making the data less informative in the first place. A complex “cat and mouse” situation emerges, with consumers attempting to “hide” as firms seek to estimate their preferences.
Our first contribution consists of uncovering that firms choose a suboptimal use of the available data to improve the quality of the underlying data. Specifically, firms can mitigate their losses if they commit to persistent scores—those that give excessive importance to past information. This may seem counterintuitive, as the long-term consequences of a very persistent score suggest consumers might become more scared of revealing information and facing high prices for a long time. But a score that overweighs the past also correlates less with current willingness to pay, so prices initially react less to changes in the score. Therefore, scores that are more persistent than those that arise in a cat and mouse world can be more profitable, because they incentivize consumers to signal more of their information.
Score “Transparency” Is Critical
Our second contribution consists of showing that the possibility of data collection benefiting consumers via lower prices relies heavily on making scores transparent. To make this point, we assess the current market paradigm whereby the score is hidden to the consumer.
When signals of purchases are imperfect, a strategic consumer will not know her score just by knowing her past behavior. But prices will convey information. Specifically, the observation of a high price today tells the consumer that firms think she has a high willingness to pay, and hence that prices will remain high in the future due to the score’s persistence. If the consumer then expects to purchase relatively few units, she is less inclined to reduce her demand due to the discount being applied to a few units only. Thus, the consumer becomes less price sensitive relative to the case in which the score is observable. (In this latter case, the consumer would be able to identify “abnormally” high prices as those above what her score dictates, enabling her to forgo bad offers.)
With a reduced sensitivity, firms make prices more responsive to the score. While this exacerbates the demand reduction and results in lower purchases, prices are nevertheless higher, which ends up hurting consumers. What is more, strategic consumers to whom scores are hidden can be worse off than their naïve counterparts. Our results can inform policy: consumer awareness of the potential for price discrimination and score transparency have complementary roles, and one without the other may be detrimental to welfare.
Alessandro Bonatti is a professor of applied economics at the MIT Sloan School of Management.

Gonzalo Cisternas is a financial research advisor in Non-Bank Financial Institution Studies in the Federal Reserve Bank of New York’s Research and Statistics Group.
How to cite this post:
Alessandro Bonatti and Gonzalo Cisternas, “Consumer Scores and Price Discrimination,” Federal Reserve Bank of New York Liberty Street Economics, July 11, 2022, https://libertystreeteconomics.newyorkfed.org/2022/07/consumer-scores-and-price-discrimination/.
Disclaimer
The views expressed in this post are those of the author(s) and do not necessarily reflect the position of the Federal Reserve Bank of New York or the Federal Reserve System. Any errors or omissions are the responsibility of the author(s).